A narrative review of risk prediction models for lung cancer screening
Introduction
Lung cancer represents the number one cause of cancer-related mortality in the United States (U.S.) in both men and women. It surpasses the combined mortality of breast, prostate and colorectal cancer. Lung cancer created an estimated 228,150 new cases in the U.S. in 2019. Worldwide, there were 2.09 million new cases and 1.76 million deaths. Overall 5-year survival is only 19.4%. However, prognosis varies dramatically with stage of diagnosis ranging from 5.2% with distant spread (stage IV), to as high as 88% 10-year survival with screened and treated stage I lung cancer (1-3). Prior to screening, 57% of all lung cancer patients already had stage IV disease when they first presented (4).
Since 2013, the U.S. Preventative Services Task Force (USPSTF) has endorsed the recommendation of low dose computed tomography (LDCT) screening in those aged 55–80 with 30-pack year smoking history who are active smokers or quit within the past 15 years with a “B” recommendation (5). The USPSTF recommendations were largely based off the well-powered randomized control National Lung Screening Trial (NLST) (6). The entry criteria of this screening trial required ages 55–74 years-old with at least 30 pack-year smoking and have smoked within the past 15 years.
Through the Affordable Care Act (ACA), the recommendation by the USPSTF guaranteed private insurance coverage for LDCT lung cancer screening up to age 65, the age at which Americans become insured by Medicare. The National Coverage Decision by the Centers for Medicaid Services (CMS) on Feb 5, 2014 established a public policy of providing Medicare insurance coverage for LDCT to screen for lung cancer in active smokers and former smokers up to age 77 years with a 30-pack year smoking history who quit less than 15 years prior (7).
Lung cancer screening guidelines had been endorsed by many professional societies including the National Comprehensive Cancer Network (NCCN), the American Association of Thoracic Surgeons (AATS), Society for Thoracic Surgeons (STS), American Lung Association (ALA), and the American Cancer Society (ACS) (8). Not all of these recommendations had been exactly alike. In particular the NCCN and the AATS recognized that some younger patients (age 50 years) and with less tobacco exposure of 20 pack years could have an additional risk factor that would place them at sufficient risk to consider LDCT screening (9,10). This had led the NCCN to classify patients into 2 groups based on presence of “additional factors” to guide screening recommendations. The desire to name these “additional factors” has led to the suggestion that risk prediction models could identify high risk patients for screening beyond the tobacco exposure alone. Meanwhile, the USPSTF has recently drafted a new grade “B” recommendations on July 7th, 2020 which would expand screening recommendation to adults ages 50–80 years old who have a 20 pack-year smoking history and currently smoke, or have quit within the past 15 years unless they develop a health problem that substantially limits life expectancy or the ability or willingness to have curative lung surgery. The final statement is expected to be published at the end of 2020 (11).
Evidence supporting lung cancer screening with LDCT for 30 pack year smokers over the age of 55 is well validated and has found additional support with the extended follow up from the International Lung Screening Trial (ILST) trial (12) and the Nederlands-Leuvens Longkanker Screening Onderzoek (NELSON) randomized control trial (13). Both of these well powered studies have validated the presence of early stage shift with screening as well as lung-cancer-specific mortality benefit associated with screening.
However, screening criteria for these landmark studies are narrow and current guidelines require a history of smoking. More attention has recently turned to the development and validation of risk-models to select for screening with the goal to (I) incorporate additional risk factors that may warrant screening to reduce lung-cancer specific mortality apart from age and smoking history, and (II) reduce the risk of false positives from screening which may lead to unwarranted invasive additional testing. Our review will hence discuss the history and current status and controversies of lung cancer risk-prediction models, as well as the pros and cons of their use in contemporary screening protocols. We present the following article in accordance with the Narrative Review reporting checklist (available at https://ccts.amegroups.com/article/view/10.21037/ccts-20-165/rc).
Methods
Search and review of the literature
Our paper serves as an expert narrative review of new literature pertaining to lung cancer risk prediction models for screening. Articles for inclusion were identified by both author’s after search of PubMed, Medline and Cochrane library were performed from date of inception through August 24, 2020. We used the MeSH search terms: “lung cancer”; “screening”; “low dose CT”, and “risk prediction model” to identify any new relevant articles for inclusion in our review. The results of these studies are summarized below.
We also checked references of relevant articles and review articles for inclusion. In addition, after consensus review between both authors, articles deemed relevant and not identified in above mentioned searches were included in the review. We finally excluded all studies: (I) that were not published in English, or (II) were case-reports or small case-series.
Review and results
What is a risk model for lung cancer screening?
A risk model is a mathematical model that predicts the risk of lung cancer within a certain cohort of patients. Although the lung cancer screening criteria limits participation to a “high risk group” of patients between ages 54 and 77 years with 30 pack years of smoking, it does not allow non-smokers to participate in screening. Yet, mathematical models exist that show that certain subgroups of non-smokers are at risk for lung cancer, and perhaps should be screened. By incorporating additional known risk factors for lung cancer screening into these models, risk models attempt to increase screen-preventable deaths, while reducing the number needed to screen (NNS) compared to risk-factor based inclusion criteria implored by the trials such as the NLST or NELSON trial (6,13) for which USPSTF guidelines are based (5). Two examples of these tools are the Liverpool Lung Project (LLP) (14) and the Prostate Lung Colon Ovary Cancer Screening Trial (PLCO) (15).
The LLP (14) used data from 579 lung cancer cases and 1,157 age- and sex-matched population based controls to generate an equation that could be used to calculate risk of lung cancer over the next 5 years for any individual based on age, sex, smoking history, family history of lung cancer, occupational exposure to asbestos, prior diagnosis of pneumonia, and prior diagnosis of malignant tumors other than lung cancer. This tool has been internally cross validated (AUC =0.70) in a mostly Caucasian population from the United Kingdom, including never and ever smokers up to 79 years of age. The LLP risk model was used in the United Kingdom Lung Screening (UKLS) trial (16). To enter this trial, subjects needed a calculation of >5% risk of developing lung cancer over the next 5 years.
The PLCO (15) lung cancer risk models derived from comparison between 38,000 smokers and 70,000 controls. One model was developed for the general population and one for smokers. Both PLCO models include age, socioeconomic status (classified by education), body mass index, family history of lung cancer, chronic obstructive pulmonary disease, recent chest X-ray, smoking status (never, former, current), pack-years smoked, smoking duration and smoking quitting time for the ever smokers’ model. The external validation for these two models were AUC of 0.841 and 0.784 respectively. This tool is applicable to a diverse population.
Risk-prediction models, furthermore, hope to narrow the impact of race and ethnic disparities in lung cancer screening (17). More recently, some risk-prediction models have attempted to incorporate blood biomarkers in their modeling as well, which include protein (pro-SFTPB, HE4, IGFBP2, LRG1); lipid (DAS), and antibody (LTF, ADCK1, STK10, TRIM10, KM2) (18).
Role of risk prediction models to explore cumulative risk
Since a risk prediction model is fundamentally an equation, it can be used to examine relationships between different exposures. For instance, what is the risk of developing lung cancer in the next 5 years of a non-smoking 75-year-old female with a positive family history as compared to a similar patient with a 30-pack year smoking history but no other risk factor? Often time non-smoking risk factors do not exist in isolation. The authors have previously demonstrated using the LLP model (the only model to estimate risk in never-smokers) (19) that cumulative risk factors in nonsmoking women may approach a cumulative risk of 7% of lung cancer over the next 5-year at age 75 which exceeds the 2.2% risk of lung cancer seen with similarly aged women with only 30 pack-year smoking history. Figure 1 depicts the findings of the study by Bravo-Iñiguez et al. (19) demonstrating the association between age and non-smoking risk factors as compared to 30 pack-year history.
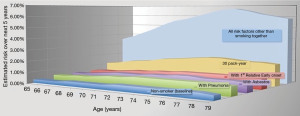
Potential Benefits for risk-prediction models in lung cancer screening
There are many pros associated with utilization of risk-models. For instance, the PLCOM2012 model compared to the NLST was noted to have had improved sensitivity (83.0% vs. 71.1%, P<0.001), higher positive predictive value (4.0% vs. 3.4%, P=0.01), and no loss of specificity (62.9% and 62.7%, respectively; P=0.54) when using absolute risk thresholds defined as: low, less than 1.0%; intermediate, 1.0% to less than 2.0%; and high, 2.0% or more (20). Similarly, the Kovalchik model found that screening the 60% at highest risk for lung cancer captured 88% of all LDCT-preventable lung cancer deaths and reduced the number needed to screen (NNS) to 161. Kovalchik et al. furthermore demonstrated that in the 20% at lowest risk of lung cancer, there were almost no cancer deaths (21). This data supports the hypothesis that with risk-models, screening could target those at highest risk while limiting unnecessary testing for the lower risk group. Temmemagi et al. combined the PLCOM2012 model with addition of the extended follow up data from the NLST trial from 2013–2018 and discovered if the initial LDCT scan was negative, that patient might actually fall below their proposed threshold for LDCT screening of 1.5% risk of developing lung cancer over the next 6 years. Some low risk patients could be dropped from a screening program with a 3-year lung cancer risk of only 0.3–0.5%. Alternatively, those with a negative screen but considered high risk by the PLCOm2012 model might warrant continued screening (22).
The number of scans (and the cost) of a lung cancer LDCT screening program is influenced by the risk-threshold cutoffs in the prediction model. For example, the PLCOm2012 study using extended NLST follow up data, found 56% of patients with a negative screen were at or above the threshold to continue screening if the 6-year risk was set at 1.5%. This dropped to 42.1% when the risk-threshold was raised to 2.0% (22).
To date, risk prediction models have had limited validations, based largely on case control studies and cohort studies or modeled after previous clinical trials. The most recent systematic review of risk-prediction model studies for lung cancer screening identified 11 multiple-use models and 17 single-use risk prediction (23). Most studies included in their analysis were case-control or cohort studies, and risk of bias was noted to be high overall. Of the 11 studies used multiple times, 6 studies were externally validated with sample sizes ranging from 325 to 44,233 cases. The accuracy of the models was tested with area under receiving curve (AUC) or C statistic. The minimum AUC of each model was 0.57 and the largest was 0.87 with the PLCO model. The smallest C statistic was 0.59 and the largest being 0.85. A summary of these models is reproduced with permission by Tang et al. (Table 1).
Table 1
Model | Year | Research design | Study design | Population | Modeling sample | AUC (95% CI) | C-index (95% CI) |
---|---|---|---|---|---|---|---|
Bach (24) | 2003 | Cohort study | Cox proportional hazards regression | Aged 50−69 years, current and former smokers | 18,172 | 0.72 | |
Spitz (25) | 2007 | Case-control study | Logistic | Never, former and current smokers | Cancer case 1,851/control 2,001 never smokers; cancer cases 330/control 379 former smokers: cancer case 784/control 884; current smokers: cancer case 737/control 738 | Never smokers, 0.57 (0.47−0.66); former smokers, 0.63 (0.58−0.69); current smokers, 0.58 (0.52−0.64) |
|
Spitz (26) | 2008 | Case-control study | Logistic | Current and former smokers, White non-Hispanic cases | Current smokers: cancer case 350/control 244; former smokers: cancer case 375/control 371 |
Former smokers, 0.70 (0.66-0.74); current smokers, 0.58 (0.52-0.64) |
Never smokers: 0.59 (0.51−0.67); former smokers: 0.63 (0.58−0.67); current smokers: 0.65 (0.60−0.69) |
LLP (14) | 2008 | Case-control study | Logistic | Aged 20−80 years | Cancer case 579/control 1,157 | 0.71 | |
LLPi (27) | 2015 | Case-control study | Cox proportional hazards regression | Aged 45−79 years | 8,760: cancer case 237, control 8,523 | 0.852 (0.831−0.873) | |
PLCO (28) | 2009 | Cohort study | Logistic | Aged 55−74 years who were free of the cancers under study | 12,314 | 0.865 | |
PLCO (15) | 2011 | Cohort study | Logistic | Aged 55−74 years, Model 1: the PLCO control arms; Model 2: smokers only | Model 1: 70,962; Model 2: 38, 254 | Model 1: 0.859 (0.8476−0.8707); Model 2: 0.809 (0.7957−0.8219) |
|
PLCOM (20) | 2012 | Cohort study | Logistic | Aged 55−74 years, former smokers | 36,286 | 0.803 (0.782−0.813) | |
Etzel (29) | 2008 | Case-control study | Logistic | African Americans | Cancer case 491/control 497 | 0.75 | |
Pittsburgh (30) | 2015 | Case-control study | Logistic | Aged 55−74 years, current and former smokers | LDCT 25,929/CXR 25,648 | LDCT 0.679/CXR 0.687 | |
Hoggart (31) | 2012 | Cohort study | Survival analysis | Aged 40−65 years, current, former and never smokers | 169,035 (90% of the data) | One year-current: 0.82; former: 0.83; never: 0.84. 5 year-current: 0.77; former: 0.72; never: 0.79 |
AUC, area under curve; C-index, concordance statistic; CI, confidence interval; CXR, chest radiograph; LDCT, low dose computed tomography; LLP, Liverpool Lung Project Model; LLPi, Liverpool Lung Project Risk Model; PLCO, prostate, lung, colorectal, and ovarian cancer screening trial; PLCOM, prostate, lung, colorectal, and ovarian cancer screening trial model.
Variables incorporated into these 11 multiple-use studies were quite heterogenous, but overall variables included 6 main aspects: sociodemographic factors, exposure history, smoking history, medical history, family history, and genetic factors.
In another review of 9 lung cancer risk models in the United States, 4 models were recently identified as performing best in selecting ever-smokers for lung screening including: the Bach model, PLCOm2012, LCRAT, and LCDRAT (32). The PLCOm2012 study is now perhaps the most validated, with the best external validation in the European Prospective Investigation in Cancer and Nutrition study (33), as well as well as in places like the Australian population-based cohort study of 95,882 participants (34).
Currently, the International Lung Screen Trial (ILST) is prospectively enrolling 4,500 participants in Canada, Australia and elsewhere based on a 6-year risk of greater than or equal to 1.5% using either PLCOm2012 or USPSTF criteria (35). While not-randomized, the ILST may serve as one of the first prospective studies attempting to validate use of risk-projection models to influence the screened study population. Furthermore, Cancer Care Ontario (CCO) has developed a lung screening pilot for high-risk individuals (HR_LCSP) with plans to enroll 3,000 individuals for annual screening over a 2-year period. Unlike the ILST, the HR_LCSPs is based on PLCOm2012 risk ≥2% over 6 years (36).
Limitations of risk-prediction models for lung cancer screening
Despite the enticing concept of risk-modeling for lung cancer screening, many of the current models to date have limitations. This is one of the main reasons why risk projection models are not universally adopted by screening programs. We have seen some adoption by professional societies such as NCCN (37) and AATS (38), which recommend screening with only 20 pack year smoking history and one other variable which increases risk of malignancy, however, this has not been formally adopted by USPSTF guidelines to date (5) nor other societal guidelines such as CHEST (39).
One major limitation of risk-prediction models for lung cancer screening include utilization of heterogenous variables. For instance, the Bach model, based on the Carotene and Retinol Efficacy trial (CARET) includes age, gender, smoking intensity, duration, years since cessation and asbestos exposure (24). Meanwhile, the LLPv2 used family history of lung cancer, prior diagnosis of malignancy other than lung cancer, prior diagnosis of pneumonia and exposure to asbestos on top of age and smoking history (14). It is also less clear whether full benefit is also observed when using abbreviated risk-prediction models (40), or whether inclusion of biomarkers (18) is beneficial or cost-effective. Furthermore, some models such as the HUNT lung cancer model (41), which is a Cox model based on Norwegian data, includes complex variables such as “daily cough”. Variables like “daily cough”, are difficult to categorize and may be an indicator of already present malignancy, thereby not targeting asymptomatic individuals, as is the goal for a lung cancer screening program.
Many risk-projection models use non-uniform risk-thresholds for screening, often ranging from a risk threshold of greater than or equal to 1.5% to 2% over 5 to 6 years. This fact is in part due to lack of societal consensus on risk thresholds to screen. For instance, the American Association of Thoracic Surgery recommends a risk threshold of ≥5% over 5 years (10) which notably varies from the 1.5% reported in the NLST trial (6). However, as evidenced by the study by Tammemagi et al. (15) using PLCOm2012, variation in risk threshold significantly changes the proportion of patients deemed high-risk for which screening would be recommended.
Furthermore, risk-models are retrospective applications of models to previously conducted lung screening cohorts or clinical trials. By using retrospective application to prior studies to model these risk-models, it often requires assumption of the benefits seen in their trial, such as those done by Katki et al. (42) or Kovalchik et al. (21) using NLST-like benefits. This raises the question as to whether conditions seen in the clinical trial are generalizable to the overall population. For instance, Chien et al. created a risk prediction model of never-smoking females in Asia, however their thresholds were based on non-smokers in North America. Therefore, without developing a new prospective cohort study to build a model, threshold and calibration is less reliable as noted by their study (33).
Additionally, real life adherence to screening with LDCT is suboptimal and hard to measure. Quantified screening adherence rates for lung cancer fall below that for other screened cancers. Colon, breast, and cervical cancer have reported rates ranging from approximately 50–90% depending on screening modality. Adherence rates from 2015 using the National Health Interview Survey (NHIS) found the LDCT lung screening rate to be a disappointing 3.9%, only 0.6% higher than in 2010 (43). The most recent study on adherence by the general US population found LDCT screening adherence to be only 14.4% (40), well below that seen in clinical trials. It has also been demonstrated that adherence to screening varies by geography, insurance status, type of center, as well as sociodemographic factors such as education, race and gender. These variations pose additional challenges to the generalizability of risk-model and established clinical trials (44-48).
Risk-projection models may additionally identify patients for LDCT screening with a greater number of comorbidities and competing causes of death. Inclusion of these patients may decrease the benefit of lung cancer screening, such as years of life gained, quality-adjusted life years gained, and deaths averted. These factors could allow screening with NLST criteria (30 pack years tobacco, age 55–74, and smoked within last 15 years) to potentially yield better outcomes. However, advocators for risk-projection models suggest that trials such as NLST included many low-risk patients who might not benefit from screening, potentially balancing this limitation to a degree (17).
Conclusions
In summary, while there is increasing evidence to support the use of risk projection modeling for lung cancer screening, the authors believe that prospective validation studies are warranted, such as those done with nodule-management classification systems such as Lung-RADS. We hope the results from the prospective non-randomized ILST currently enrolling based on PLCOm2012 criteria may provide more data for further refinement. Until that is done, we acknowledge uncertainty exists about the generalizability of these models. We feel they are helpful for identifying other risk factors for the NCCN Group 2 patients (as supported by the new USPSTF recommendations recently open for public comment). We think it highly likely that risk prediction models will be incorporated into screening guidelines for all cancers within the next 25 years.
Acknowledgments
Funding: None.
Footnote
Provenance and Peer Review: This article was commissioned by the Guest Editor Sandra Starnes for the series “Lung Cancer Screening” published in Current Challenges in Thoracic Surgery. The article has undergone peer review.
Reporting Checklist: The authors have completed the Narrative Review reporting checklist. Available at https://ccts.amegroups.com/article/view/10.21037/ccts-20-165/rc
Conflicts of Interest: Both authors have completed the ICMJE uniform disclosure form (available at https://ccts.amegroups.com/article/view/10.21037/ccts-20-165/coif). The series “Lung Cancer Screening” was commissioned by the editorial office without any funding or sponsorship. The authors have no other conflicts of interest to declare.
Ethical Statement: The authors are accountable for all aspects of the work in ensuring that questions related to the accuracy or integrity of any part of the work are appropriately investigated and resolved.
Open Access Statement: This is an Open Access article distributed in accordance with the Creative Commons Attribution-NonCommercial-NoDerivs 4.0 International License (CC BY-NC-ND 4.0), which permits the non-commercial replication and distribution of the article with the strict proviso that no changes or edits are made and the original work is properly cited (including links to both the formal publication through the relevant DOI and the license). See: https://creativecommons.org/licenses/by-nc-nd/4.0/.
References
- Lung Cancer Facts: 29 Statistics and Facts | LCFA. [Online] Lung Cancer Foundation of America. Available online: https://lcfamerica.org/lung-cancer-info/lung-cancer-facts/ [Accessed: 21st January 2020].
- Cancer of the Lung and Bronchus - Cancer Stat Facts. [Online] SEER. Available online: https://seer.cancer.gov/statfacts/html/lungb.html [Accessed: 21st January 2020].
- International Early Lung Cancer Action Program Investigators. Survival of patients with stage I lung cancer detected on CT screening. N Engl J Med 2006;355:1763-71. [Crossref] [PubMed]
- Cancer Facts & Figures 2020 | American Cancer Society. [Online] Available online: https://www.cancer.org/research/cancer-facts-statistics/all-cancer-facts-figures/cancer-facts-figures-2020.html [Accessed: 30th September 2020].
- Recommendation: Lung Cancer: Screening | United States Preventive Services Taskforce. [Online] Available online: https://www.uspreventiveservicestaskforce.org/uspstf/recommendation/lung-cancer-screening [Accessed: 30th September 2020].
- National Lung Screening Trial Research Team. Reduced lung-cancer mortality with low-dose computed tomographic screening. N Engl J Med 2011;365:395-409. [Crossref] [PubMed]
- Lung Cancer Screening Coverage. [Online] Available online: https://www.medicare.gov/coverage/lung-cancer-screenings [Accessed: 30th September 2020].
- Wender R, Fontham ETH, Barrera E, et al. American Cancer Society lung cancer screening guidelines. CA Cancer J Clin 2013;63:107-17. [Crossref] [PubMed]
- NCCN Clinical Practice Guidelines in Oncology. [Online] Available online: https://www.nccn.org/professionals/physician_gls/default.aspx#site [Accessed: 30th September 2020].
- Jaklitsch MT, Jacobson FL, Austin JHM, et al. The American Association for Thoracic Surgery guidelines for lung cancer screening using low-dose computed tomography scans for lung cancer survivors and other high-risk groups. J Thorac Cardiovasc Surg 2012;144:33-8. [Crossref] [PubMed]
- Draft Recommendation: Lung Cancer: Screening | United States Preventive Services Taskforce. [Online] Available online: https://www.uspreventiveservicestaskforce.org/uspstf/draft-recommendation/lung-cancer-screening-2020 [Accessed: 30th September 2020]
- National Lung Screening Trial Research Team. Lung Cancer Incidence and Mortality with Extended Follow-up in the National Lung Screening Trial. J Thorac Oncol 2019;14:1732-42. [Crossref] [PubMed]
- de Koning HJ, van der Aalst CM, de Jong PA, et al. Reduced Lung-Cancer Mortality with Volume CT Screening in a Randomized Trial. N Engl J Med 2020;382:503-13. [Crossref] [PubMed]
- Cassidy A, Myles JP, van Tongeren M, et al. The LLP risk model: an individual risk prediction model for lung cancer. Br J Cancer 2008;98:270-6. [Crossref] [PubMed]
- Tammemagi CM, Pinsky PF, Caporaso NE, et al. Lung Cancer Risk Prediction: Prostate, Lung, Colorectal and Ovarian Cancer Screening Trial Models and Validation. JNCI 2011;103:1058-68. [Crossref] [PubMed]
- McRonald FE, Yadegarfar G, Baldwin DR, et al. The UK Lung Screen (UKLS): demographic profile of first 88,897 approaches provides recommendations for population screening. Cancer Prev Res (Phila) 2014;7:362-71. [Crossref] [PubMed]
- Tammemägi MC. Selecting lung cancer screenees using risk prediction models—where do we go from here. Transl Lung Cancer Res 2018;7:243-53. [Crossref] [PubMed]
- Feng Z, Hanash S, Tammemagi M. Incorporating Biomarkers to Improve Lung Cancer Risk Prediction. Available online: https://grantome.com/grant/NIH/U01-CA194733-02 [Accessed: 30th September 2020].
- Bravo-Iñiguez CE, Fox SW, De Leon LE, et al. Cumulative nonsmoking risk factors increase the probability of developing lung cancer. J Thorac Cardiovasc Surg 2019;158:1248-1254.e1. [Crossref] [PubMed]
- Tammemägi MC, Katki HA, Hocking WG, et al. Selection criteria for lung-cancer screening. N Engl J Med 2013;368:728-36. [Crossref] [PubMed]
- Kovalchik SA, Tammemagi M, Berg CD, et al. Targeting of Low-Dose CT Screening According to the Risk of Lung-Cancer Death. N Engl J Med 2013;369:245-54. [Crossref] [PubMed]
- Development and Validation of a Multivariable Lung Cancer Risk Prediction Model That Includes Low-Dose Computed Tomography Screening Results: A Secondary Analysis of Data From the National Lung Screening Trial | Cancer Screening, Prevention, Control | JAMA Network Open | JAMA Network. [Online] Available online: https://jamanetwork.com/journals/jamanetworkopen/fullarticle/2726714 [Accessed: 30th September 2020].
- Tang W, Peng Q, Lyu Y, et al. Risk prediction models for lung cancer: Perspectives and dissemination. Chinese Journal of Cancer Research 2019;31:316-28. [Crossref] [PubMed]
- Bach PB, Kattan MW, Thornquist MD, et al. Variations in lung cancer risk among smokers. J Natl Cancer Inst 2003;95:470-8. [Crossref] [PubMed]
- Spitz MR, Hong WK, Amos CI, et al. A risk model for prediction of lung cancer. J Natl Cancer Inst 2007;99:715-26. [Crossref] [PubMed]
- Spitz MR, Etzel CJ, Dong Q, et al. An expanded risk prediction model for lung cancer. Cancer Prev Res (Phila) 2008;1:250-4. [Crossref] [PubMed]
- Marcus MW, Chen Y, Raji OY, et al. LLPi: Liverpool Lung Project Risk Prediction Model for Lung Cancer Incidence. Cancer Prev Res (Phila) 2015;8:570-5. [Crossref] [PubMed]
- Tammemagi MC, Freedman MT, Pinsky PF, et al. Prediction of true positive lung cancers in individuals with abnormal suspicious chest radiographs: a prostate, lung, colorectal, and ovarian cancer screening trial study. J Thorac Oncol 2009;4:710-21. [Crossref] [PubMed]
- Etzel CJ, Kachroo S, Liu M, et al. Development and validation of a lung cancer risk prediction model for African-Americans. Cancer Prev Res (Phila) 2008;1:255-65. [Crossref] [PubMed]
- Wilson DO, Weissfeld J. A simple model for predicting lung cancer occurrence in a lung cancer screening program: The Pittsburgh Predictor. Lung Cancer 2015;89:31-7. [Crossref] [PubMed]
- Hoggart C, Brennan P, Tjonneland A, et al. A Risk Model for Lung Cancer Incidence. Cancer Prev Res (Phila) 2012;5:834-46. [Crossref] [PubMed]
- Katki HA, Kovalchik SA, Petito LC, et al. Implications of Nine Risk Prediction Models for Selecting Ever-Smokers for Computed Tomography Lung Cancer Screening. Ann Int Med 2018;169:10-9. [Crossref] [PubMed]
- Chien LH, Chen CH, Chen TY, et al. Predicting Lung Cancer Occurrence in Never-Smoking Females in Asia: TNSF-SQ, a Prediction Model. Cancer Epidemiol Biomarkers Prev 2020;29:452-9. [Crossref] [PubMed]
- Weber M, Yap S, Goldsbury D, et al. Identifying high risk individuals for targeted lung cancer screening: Independent validation of the PLCOm2012 risk prediction tool. Int J Cancer 2017;141:242-53. [Crossref] [PubMed]
- Lim KP, Marshall H, Tammemägi M, et al. Protocol and Rationale for the International Lung Screening Trial. Ann Am Thorac Soc 2020;17:503-12. [Crossref] [PubMed]
- Determination of the Eligibility Criteria for Cancer Care Ontario’s Lung Cancer Screening Pilot for People at High Risk | A98. CLINICAL STRATEGIES TO IMPROVE LUNG CANCER EARLY DETECTION: WHO IS AT RISK HERE? [Online] Available online: https://www.atsjournals.org/doi/abs/10.1164/ajrccm-conference.2018.197.1_MeetingAbstracts.A2472 [Accessed: 30th September 2020].
- Wood DE, Kazerooni EA, Baum SL, et al. Lung Cancer Screening, Version 3.2018, NCCN Clinical Practice Guidelines in Oncology. J Natl Compr Canc Netw 2018;16:412-41. [Crossref] [PubMed]
- Lung Cancer Assessment Tool - AATS.org. [Online] Available online: https://www.aats.org/AATSIMIS/iParts/Custom/Controls/LungCancerScreening/lungcancer.html [Accessed: 30th September 2020]
- Mazzone PJ, Silvestri GA, Patel S, et al. Screening for Lung Cancer: CHEST Guideline and Expert Panel Report. Chest 2018;153:954-85. [Crossref] [PubMed]
- Zahnd WE, Eberth JM. Lung Cancer Screening Utilization: A Behavioral Risk Factor Surveillance System Analysis. Am J Prev Med 2019;57:250-5. [Crossref] [PubMed]
- Markaki M, Tsamardinos I, Langhammer A, et al. A Validated Clinical Risk Prediction Model for Lung Cancer in Smokers of All Ages and Exposure Types: A HUNT Study. EBioMedicine 2018;31:36-46. [Crossref] [PubMed]
- Katki HA, Kovalchik SA, Berg CD, Cheung LC, Chaturvedi AK. Development and Validation of Risk Models to Select Ever-Smokers for CT Lung Cancer Screening. JAMA 2016;315:2300-11. [Crossref] [PubMed]
- Jemal A, Fedewa SA. Lung Cancer Screening With Low-Dose Computed Tomography in the United States-2010 to 2015. JAMA Oncol 2017;3:1278-81. [Crossref] [PubMed]
- Cattaneo SM, Meisenberg BR, Geronimo MCM, et al. Lung Cancer Screening in the Community Setting. Ann Thorac Surg 2018;105:1627-32. [Crossref] [PubMed]
- Eberth JM, Bozorgi P, Lebrón LM, et al. Geographic Availability of Low-Dose Computed Tomography for Lung Cancer Screening in the United States, 2017. Prev Chronic Dis 2018;15:E119. [Crossref] [PubMed]
- Begnaud A, Hall T, Allen T. Lung Cancer Screening With Low-Dose CT: Implementation Amid Changing Public Policy at One Health Care System. American Society of Clinical Oncology Educational book. American Society of Clinical Oncology. Annual Meeting. 2016;35:e468-75.
- Clarke TC, Soler-Vila H, Fleming LE, et al. Trends in Adherence to Recommended Cancer Screening: The US Population and Working Cancer Survivors. Front Oncol 2012;2:190. [Crossref] [PubMed]
- Schütte S, Dietrich D, Montet X, et al. Participation in lung cancer screening programs: are there gender and social differences? A systematic review. Public Health Rev 2018;39:23. [Crossref] [PubMed]
Cite this article as: Dezube AR, Jaklitsch MT. A narrative review of risk prediction models for lung cancer screening. Curr Chall Thorac Surg 2023;5:3.