Radiographic assessment of small lung nodules: what can we do and what information does it give us?
Introduction
Radiographic assessment of small pulmonary nodules is a frequently encountered clinical dilemma; at any given time, a large number of nodules are being longitudinally followed. The key question of whether a given nodule represents a malignant lesion is of paramount importance and can result in an optimal treatment strategy. The assessment comprises of anatomical and functional information, which when combined with demographic and clinical parameters can help with risk stratification, and lead to development of an effective treatment option based on the initial presentation (1).
The Fleischner society defines a pulmonary nodule as a “rounded opacity, well or poorly defined, measuring up to 3 cm in diameter”, and surrounded by aerated lung, with nodular opacities <3 mm referred to as micronodules (2). Small nodules are those that have an average of the long and short-axis diameters of <10 mm (3,4). With the widespread use of multidetector computed tomography (CT), there has been a significant increase in discovery of these small pulmonary nodules, which pose a significant diagnostic burden (5). These are not visible on chest radiographs and are too small to be reliably characterized on positron emission tomography (PET) or magnetic resonance imaging (MRI). Radiographic assessment includes qualitative and quantitative functional evaluation which can be combined with temporal evolution to determine the malignant potential and risk stratification. In this article, the role of imaging in the characterization of small nodules is examined and the key information that can be extracted from each modality for optimal triage is presented.
Qualitative and quantitative assessment
Radiographic assessment at initial discovery comprises of a qualitative assessment based on shape, density, calcification, location, and ancillary findings which can then be combined with quantitative parameters such as size, standard uptake value (SUV) on PET, apparent diffusion coefficient (ADC) on MRI, perfusion parameters derived from dynamic enhanced CT or MRI to non-invasively characterize the nodule. CT forms the main workhorse of evaluation and can be augmented by PET/CT or MRI to further characterize in specific circumstances.
For optimal radiographic assessment, image acquisition and reconstruction techniques are standardized, and the current recommendations require CT images to be reconstructed with contiguous thin slices (<3 mm, ideally <1 mm), with multi-planar reformats (MPRs) to help differentiate between nodule and scarring or atelectasis (4). Thicker section reconstructions (5 mm or greater) increase partial volume averaging which reduces the sensitivity for small nodule detection, and limits characterization. Post processing including volumetric assessment also requires thin section data acquired in soft tissue kernel. The use of intravenous iodinated contrast on CT scans is not required for pulmonary nodule evaluation; a few research studies have explored it to differentiate benign from malignant nodules, but the technique has not been found to be practical and feasible due to low positive predictive value and higher radiation exposure (6).
The Fleischner society recommends that nodule measurements should be recorded to the nearest millimeter by averaging the long- and short-axis diameters measured on the same axial, coronal, or sagittal plane using electronic calipers or semiautomated methods on the image plane depicting the largest nodule size (3,4). Recent international guideline iterations have introduced nodule volume as a surrogate of size, based mainly on data from the recently reported Dutch-Belgian Lung Cancer Screening trial (NELSON) (7). The British Thoracic Society (BTS) guidelines also incorporates initial nodule volume and volume doubling time (VDT) into their follow-up recommendations (8).
Nodule size is an important predictor of malignancy. The analysis of cohorts derived from the Pan-Canadian Early Detection of Lung Cancer Study (PanCan) and chemoprevention trials at the British Columbia Cancer Agency (BCCA), demonstrated that nodule size (maximal nodule diameter) had a significant nonlinear relationship in predicting likelihood of lung cancer (9). The prevalence of lung malignancy in subjects with small nodules (<6 and <5 mm) in the National Lung Screening Trial (NLST) and NELSON trials was extremely low at 0.5% and 0.6% respectively (10,11). The updated Fleischner society guidelines also do not recommend routine CT follow-up for patients with solid nodules <6 mm (<100 mm3), except for those with suspicious morphology, upper lobe location, or patients with high clinical risk, in which a follow-up at 12 months may be considered (4). Similarly, the BTS guidelines do not advocate routine follow-up for solid nodules less than 5 mm in diameter or less than 80 mm3 in volume (8). For solitary noncalcified solid nodules measuring 6–8 mm (100–250 mm3), the Fleischner guidelines recommend CT follow-up at 6–12 months, and with potential further follow-up at 18–24 months for high-risk patients or nodule with more concerning morphology (4). Nodules in this size range have an average malignancy risk of 0.5–2.0% based on data from various lung cancer screening trials, justifying the recommendation for follow-up (9,11). Solitary noncalcified nodules >8 mm (>250 mm3) are at higher risk for malignancy, and are recommended to undergo a 3-month CT follow-up, PET/CT, tissue sampling, or a combination of these methods depending on patient and nodule factors (4). For patients with multiple solid nodules, management should be based on the dominant, most suspicious nodule, which may or may not be the largest. When multiple non-calcified nodules less than 6 mm in size are present, routine follow-up is not required in the absence of a known malignancy or risk factors; these small nodules usually represent healed granulomata or benign intrapulmonary lymph nodes. If one of these nodules is 6 mm or larger in size, follow-up is recommended, initially at 3–6 months as metastases are a concern and particularly if the nodules have a peripheral and/or lower zone predominance and vary in size (4). In most cases, metastases will demonstrate growth on the 3–6 months interval CT, except in certain slow growing cancers such as metastatic papillary thyroid cancer.
Nodule density is an important consideration when assessing risk of malignancy. In general, nodules can be divided into solid and subsolid nodules (SSNs), with the latter incorporating both pure ground-glass nodules (GGNs) and part-solid nodules (PSNs). The key features to assess for in evaluation/surveillance of SSNs is interval growth and/or development of a solid component; the presence of a definite measurable solid component in a SSN evaluated on a mediastinal/soft tissue window is highly concerning for invasive adenocarcinoma, with a reported specificity ranging from 0.86–0.96 (12). An interval of 3–4 years is required, on average, to demonstrate growth/develop an invasive adenocarcinoma in pure GGNs. Lee et al. (13) reported a series of 235 SSNs >6 mm that were stable for more than 5 years. They followed up these nodules for a median of 9 years after the initial 5-year period, with only 2% of SSNs demonstrating growth, suggesting that 5-year stability marks an appropriate end-point for routine CT follow-up for SSNs (14). Per Fleischner criteria, routine follow-up is not recommended for solitary pure GGNs <6 mm in the absence of other risk factors; for pure GGNs >6 mm, it is currently recommended to follow up to 5 years as they may represent indolent or slow growing adenocarcinoma spectrum lesions (4). For solitary PSNs measuring 6 mm or larger, measurement of the size of the solid component is important in determining the risk of malignancy. PSNs with a solid component less than 6 mm typically represent either adenocarcinoma in situ or minimally invasive adenocarcinomas, with a solid component of >5 mm in PSN strongly associated with local invasion (15,16). When multiple SSNs are present, infection is a consideration, particularly if the SSNs are <6 mm in size. A follow-up CT is usually recommended in 6 months to assess for resolution in these cases. For patients with multiple SSNs with at least one nodule >6 mm, management should be guided by the most suspicious appearing nodule, with multifocal adenocarcinoma spectrum lesions a consideration if they persist on the 3–6 months follow-up CT.
Interobserver agreement by radiologists in classifying nodules into solid or subsolid categories is only moderate at 0.58, with disagreement on the presence and size of a solid component in SSNs the main discrepancy (17). The updated Fleischner guidelines recommend that nodules rendered partially invisible when the sharp filter lung kernel images are viewed on a mediastinal (soft tissue) window can be classified as subsolid, and that any visible nodular component other than normal bronchovascular structures can be classified as a solid component (4). Some small solid/semisolid components may only be visible on lung windows but are of importance as they could represent early signs of invasive tumor or adenocarcinoma.
Other morphological factors such as margins and contour can also be helpful in assessing risk in small pulmonary nodules. Benign nodules typically have a well-defined margin and smooth contour, with malignant nodules showing spiculated margins and an irregular or lobulated contour. The presence of spiculation at the margins of the nodule, which is felt to represent infiltration of malignant cells along the pulmonary interstitium surrounding the nodule (18), is strongly associated with malignancy (9). It is not however pathognomonic for malignancy, and may also be seen in certain benign conditions, including lipoid pneumonia, tuberculosis (TB), and progressive massive fibrosis (18). An irregular or lobulated contour is also associated with malignancy and results from differential growth within the lesion (Figure 1). The presence on CT of a rim of ground glass surrounding a solid nodule in the center is called the halo sign, and is typically associated with fungal pneumonias, particularly invasive pulmonary aspergillosis, where the ground glass halo is felt to represent hemorrhage (19). This sign, however, is not pathognomonic for fungal infection, and may be seen occasionally in lepidic pulmonary adenocarcinoma, Kaposi sarcoma, and metastases from choriocarcinoma, angiosarcoma, and pancreatic cancer (18). The converse of this is the reverse halo or atoll sign, where there is a central area of ground glass attenuation surrounded by a halo of consolidation; this was first described in relation to cryptogenic organizing pneumonia (COP) and has been recently described as a typical CT pattern in the COVID-19 viral pneumonia (20).
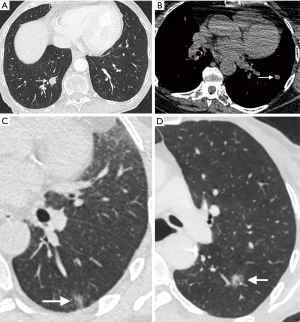
Uniformly calcified solid nodules can be categorized as granulomas, obviating the need for routine CT follow-up, and the presence of macroscopic fat within a nodule denotes that it is a benign hamartoma (Figure 1). Quantitative assessment of the density of the nodule [in Hounsfield units (HU)] can aid this assessment and should be performed on a soft-tissue window without a sharp edge-enhanced kernel to reduce the impact of beam hardening (4). Typically, fat attenuation is –40 to –120 HU and is present in up to 50% hamartomas (21). The presence of calcification within a solid nodule is best examined on a non-contrast CT reconstructed with thin sections with a smooth/soft tissue reconstruction kernel. Diffuse, bull’s-eye (central) and laminated patterns of calcification are seen in benign granulomas, with popcorn calcification characteristic of chondroid calcification in a pulmonary hamartoma. Not all calcified nodules are benign; metastases from osteosarcomas and chondrosarcomas may demonstrate these patterns of calcification, and punctate, eccentric, and amorphous calcification may be seen in primary lung cancers, particularly mucinous adenocarcinomas (18).
Cavitation within a small nodule is seen as a lucency or low-attenuation within the nodule, and may be seen in infection such as TB, vasculitis, inflammatory etiologies as well as malignancy (2). Both primary and metastatic cancers may show cavitation, especially squamous cell cancers; central cavitation in a metastasis is also seen as a treatment effect of chemotherapy. The thicker and more irregular the cavity wall, the greater chance of malignancy, with up to 95% of cavitary nodules with a wall thickness of greater than 15 mm reported as malignant (22). But this does not by definition apply to small pulmonary nodules which demonstrate cavitation, which can make characterization challenging. The Cheerio sign relates to small pulmonary nodules with a central lucent cavity that represents a patent bronchus and is due to cellular proliferation around the patent airway. This may be due to pulmonary adenocarcinoma, pulmonary Langerhans cell histiocytosis (PLCH), or pulmonary meningothelial-like nodules; a rare entity representing proliferation of benign spindle cells (23,24). Central, bubble-like lucencies within a nodule, often referred to as pseudocavitation, are associated with adenocarcinomas particularly and are thought to represent growth of tumor cells around normal aerated lung (Figure 1) (2,25).
Nodule location is another factor which can be useful, with an upper lobe nodule location a confirmed risk factor for lung cancer (9). Axial distribution is also a factor, with metastases and adenocarcinomas tending to be located in the periphery of the lung, while squamous cell carcinomas are more centrally located (26). Perifissural nodules (PFNs) describe small solid nodules adjacent to the pleural fissures with an oval or lentiform morphology and linear subpleural tail; they generally represent benign juxta-pleural lymph nodes which do not require routine CT surveillance (4). PFNs are frequent occurrences and accounted for approximately 20% of nodules detected in the NELSON lung cancer screening trial; some nodules demonstrated serial growth, but, reassuringly, none of them were found to be malignant on follow-up (27). There is at best only moderate interobserver agreement for classifying small solid nodules as PFNs, particularly when there are atypical features such as no visible attachment to a fissure, upper lobe nodule location, and a spiculated border (28). Caution should therefore be exercised when apparent PFNs display some atypical features, or when there is a history of malignancy, and a surveillance CT should be considered.
The presence of background lung disease, especially emphysema, has been demonstrated as an independent risk factor for lung malignancy. The presence of emphysema on CT is a strong independent risk factor for lung cancer, with an odds ratio of 3.1 in a high-risk cohort with a history of tobacco exposure (29). This is corroborated by data from the NLST, which demonstrated that the presence of emphysema on CT is an independent risk factor for malignancy (30). Pulmonary fibrosis, particularly idiopathic pulmonary fibrosis, is another independent risk factor for malignancy, with a higher reported risk of lung cancer than patients with emphysema (31). Lung cancers in patients with chronic interstitial fibrosis are often difficult to diagnose due to background fibrotic changes and architectural distortion. Approximately two-thirds of lung tumors in these patients are found at the interface between normal and fibrotic lung, and can often display unusual morphology, such as a bandlike appearance or stellate shape (32).
Functional assessment
Functional imaging can be combined with anatomic assessment with 2-deoxy-2-[18F]fluoro-D-glucose (FDG) PET-CT to help stratify malignant risk in pulmonary nodules, improving diagnostic accuracy (Figure 2) (33,34). According to the Fleischner guidelines, PET should be considered for the further assessment of malignant risk in nodules >8 mm in size (>250 mm3 volume) (4). The BTS guidelines recommend its use in patients with a greater than 10% risk of malignancy following assessment with the Brock risk prediction calculator (8). Following FDG PET-CT, the risk of malignancy can be quantified using the Herder model, which incorporates the degree of FDG uptake within a nodule (absent, faint, moderate, or intense), along with other clinico-radiological parameters (age, smoking status, history of extra-thoracic cancer, nodule size, location, and spiculations) (35). The incorporation of FDG uptake adds incremental benefit to diagnostic accuracy of the model, increasing the area under the receiver operating characteristic (ROC) curve (AUC) from 0.79 to 0.92 (35), and has been validated in a separate cohort of 240 patients (36).
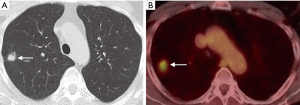
There are however a number of limitations to the use of PET that should be considered, especially in the evaluation of small nodules. In order for a nodule to be identifiable, its level of tracer uptake needs to be clearly visible above background activity. PET-CT scanners have a finite ability to resolve small objects due to limitations from the spatial resolution of the system and radiotracer kinetics, with a spatial resolution of approximately 8 mm. This means that tracer uptake in lesions smaller than this may be underestimated due to partial volume averaging, limiting its diagnostic accuracy for these nodules. There are novel PET reconstruction algorithms (Q.Clear, GE Healthcare, Chicago, IL, USA) which have been shown to increase the apparent level of FDG uptake in small lesions compared to the standard Ordered Subset Expected Maximisation (OSEM) PET reconstruction method (37), however this has not yet translated into improved diagnostic accuracy (38). The role of PET in the evaluation of sub-solid nodules is also limited; distinguishing benign from malignant SSNs on FDG PET-CT is not reliable as well-differentiated adenocarcinomas may demonstrate none or only faintly increased radiotracer uptake compared to background lung parenchyma (39). FDG is not a malignancy specific tracer, and false positive uptake may be demonstrated in a variety of inflammatory and infectious conditions, such as bacterial pneumonia, TB, and sarcoidosis. False negatives may also occur in certain cancers which do not avidly take up FDG, particularly some neuroendocrine tumors. For example, typical carcinoid tumors are generally better evaluated with somatostatin receptor analogue imaging, such as Gallium68 DOTATATE PET-CT rather than with FDG PET-CT (Figure 3) (40).
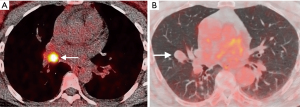
MRI has been used to differentiate benign from malignant nodules using techniques such as diffusion weighted imaging (DWI) and dynamic contrast enhanced MRI (DCE-MRI) (Figure 4). DWI-MRI sequences have been shown to be of value in differentiating benign from malignant nodules (41), but its applicability to small nodules <1 cm is uncertain and is prone to respiratory artifact limiting adequate characterization (42). Perfusion kinetics derived from DCE-MRI can help differentiate benign from malignant lesions (43). The role of MRI in the evaluation of small pulmonary nodules remains mainly in the research field for now, but promising technical developments may point to MRI playing a more central role in the future by combining anatomical and functional assessment without the exposure of ionizing radiation.
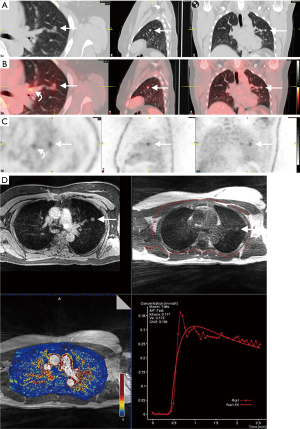
Temporal evolution and risk prediction
Assessment of nodule growth is the key reason behind performing follow-up CTs for indeterminate small pulmonary nodules. Cancers demonstrate different growth rates depending on tumor histology and grade, and the time periods suggested for surveillance are designed to both minimize the number of CTs and reduce the chance of a cancer progressing in stage during the surveillance interval. The ability to detect an increase in size of a nodule during these follow-up CTs is therefore of paramount importance. Although the current iteration of the Fleischner society guidelines recommend linear diameter measurements as the current standard of practice to assess nodule growth (4), the BTS guidelines have advocated more for semiautomated nodule volumetry, as this may be a more sensitive assessment of nodule growth (8). This difference in approach is largely due to the lack of widespread availability for robust and validated software for semiautomated volumetric nodule segmentation, although this will likely change in the future (44). VDT (measured in days) for solid malignancy is established as a robust method of growth assessment, with a doubling in volume corresponding to an approximate 25% increase in diameter (for a spherical nodule) (45). Most lung cancers will demonstrate a VDT of less than 600 days; in the NELSON trial, which primarily used nodule volume rather than diameter, small solid nodules in the 100–300 mm3 category with VDT of less than 400 days, 400–600 days, and greater than 600 days were associated with lung cancer rates of 10%, 4%, and 0.8% respectively (11). Subsolid cancerous nodules, which are nearly always adenocarcinomas, demonstrate more indolent growth, with an average VDT in the range of 3–5 years (46). These nodules however are much harder to segment accurately using current post-processing software, which makes performing accurate volumetry challenging (45). Segmentation of the solid component of PSNs has been proposed as a potential solution, although further validation is required (47).
The ultimate aim of small nodule evaluation is to estimate the individual risk of malignancy, with accurate assessment of nodule size, density, location, and morphology on CT important factors. Nevertheless, it is critical to consider clinical risk factors, such as age, gender, smoking history, and family history of lung cancer, in order to formulate a more accurate individualized clinico-radiological risk. These factors have been incorporated into several nodule risk prediction calculators designed to aid clinical decision making. There are several potential advantages to the use of these risk prediction models. Firstly, they enable an estimate of malignancy risk based upon several established clinical and imaging predictors of malignancy rather than sole reliance on a single imaging parameter. Secondly, this estimation of risk helps guide management strategies with the lowest risk favoring the least invasive approach, and such practice is supported by data from cost-effectiveness studies (48,49). They may also facilitate shared decision making between clinicians and patients by helping interpret nodule malignancy risk in the clinical context of risk of potential complications associated with any proposed intervention, such as image-guided biopsy.
The Swenson model calculates a nodule malignancy risk based on nodule parameters (diameter, spiculation, upper lobe location) and patient factors (age, smoking history, cancer history) (50). The multifactorial Brock model is derived from two Canadian lung cancer screening trials and incorporates CT findings (nodule size, type, location, count, spiculation, emphysema) along with clinical parameters (age, gender, family history of lung cancer) to derive a malignancy risk (9). This model has demonstrated good discriminatory ability, even for small nodules, and has been recommended for use by the 2015 BTS nodule guidelines; a malignancy risk of 10% has been proposed as the cut-off between surveillance and further investigation (8). In fact, it appears that these pulmonary nodule risk calculators are best suited to small nodules <10 mm and may be of more limited use in larger nodules (51). The 2017 Fleischner guidelines adapted the American College of Chest Physicians (ACCP) 2013 guidance for risk, dividing patients into low (<5%) and high (>10%) risk groupings based on a combination of nodule factors (size, spiculation, upper lobe location) and clinical features (older age, heavy smoking) (4). This distinction is based on more of a clinical impression combining these various clinic-radiological features rather than a quantitative multifactorial model with prespecified absolute thresholds (44).
Radiomics, artificial intelligence (AI), machine learning and computer aided diagnosis (CAD)
CAD can help improve detection of pulmonary nodules, and when combined with other techniques such as dual energy and temporal subtraction can help radiologists differentiate benign from malignant nodules (52). Novel data extraction and post processing methods such as radiomics, AI, and machine learning are increasingly being used to evaluate large data sets with the hope of developing algorithms that can improve radiologists’ clinical workflow. A computerized feature-based analysis of lung nodules has the potential to reliably discriminate malignant from benign lesions. Radiomics uses high-throughput computing to extract innumerable quantitative features from diagnostic images which can be of diagnostic utility (53). Studies examining these radiomics features, especially those focused on the shape and texture of a nodule are amongst the most promising in the field of nodule evaluation. Dennie et al. (54) and Kido et al. (55) demonstrated that both radiomic textural and intrinsic shape features can discriminate malignant from benign nodules. Orooji et al. (56) examined the performance of a machine learning classifier tool derived from key shape and textural features to discriminate adenocarcinomas from granulomas in a cohort of 195 nodules; the machine learning classifier tool had an AUC of 77.8%, higher than expert thoracic radiologist and pulmonologist readers. There is an emerging body of work in using radiomics to differentiate invasive from non-invasive adenocarcinoma spectrum lesions in order to triage patients with ground glass and part solid nodules to actionable strategies (57-61). Radiomics tools will almost certainly play an important role in pulmonary nodule evaluation in the future, but how and when these advanced image analysis techniques make the jump from to routine clinical practice is as yet unclear. Further integration with more advanced machine learning techniques, such as deep learning (DL), will likely be involved.
DL is a form of machine learning that uses artificial neural networks with multiple hidden layers. Advances in computational power have accelerated the development of DL applications in pulmonary image analysis, including lung nodule detection and assessment. Recent work by Cui et al. (62) demonstrates that DL is highly consistent with expert radiologists in lung nodule identification. This is an important potential application of DL as an assistant to the radiologist, particularly in high volume settings such as in lung cancer screening CT programmes, DL also has potential applications in lung nodule characterization. The Google research group recently developed a DL algorithm for detection and prediction of malignancy in lung nodules by comparing current and prior CT images from the same patient using the NLST data. The proposed Lung Malignancy Score (LUMAS) model outperformed expert radiologists and was able to reduce the false positive rate and false negative risk to 11% and 5%, respectively (63). Huang et al. (64) also developed a DL algorithm based on NLST data and used the PanCan study as its validation cohort; their “DeepLR’ algorithm demonstrated good discrimination of malignant from benign nodules, with a 3-year time-dependent AUC for cancer diagnosis of 0.89. These DL techniques are promising, however concerns remain about inconsistent validation and the widespread generalizability of these results. Thus, robust external validation will be needed in order to overcome issues related to reproducibility before translation to clinical care.
Conclusions
Careful examination of the radiological features pertaining to small pulmonary nodules improves patient care, allowing clinicians to make accurate and evidence-based management decisions. A continued multidisciplinary approach to decision making in this common, challenging scenario, encompassing thoracic radiologists, pulmonologists, surgeons and other relevant specialties is important to ensure optimal patient care.
Acknowledgments
Funding: None.
Footnote
Provenance and Peer Review: This article was commissioned by the Guest Editors (Scott Swanson, Daniel Dolan) for the series “How to Evaluate, Diagnose and Treat Small Lung Nodules” published in Current Challenges in Thoracic Surgery. The article has undergone external peer review.
Conflicts of Interest: Both authors have completed the ICMJE uniform disclosure form (available at https://ccts.amegroups.com/article/view/10.21037/ccts-20-117/coif). The series “How to Evaluate, Diagnose and Treat Small Lung Nodules” was commissioned by the editorial office without any funding or sponsorship. The authors have no other conflicts of interest to declare.
Ethical Statement: The authors are accountable for all aspects of the work in ensuring that questions related to the accuracy or integrity of any part of the work are appropriately investigated and resolved.
Open Access Statement: This is an Open Access article distributed in accordance with the Creative Commons Attribution-NonCommercial-NoDerivs 4.0 International License (CC BY-NC-ND 4.0), which permits the non-commercial replication and distribution of the article with the strict proviso that no changes or edits are made and the original work is properly cited (including links to both the formal publication through the relevant DOI and the license). See: https://creativecommons.org/licenses/by-nc-nd/4.0/.
References
- Gould MK, Fletcher J, Iannettoni MD, et al. Evaluation of patients with pulmonary nodules: when is it lung cancer?: ACCP evidence-based clinical practice guidelines (2nd edition). Chest 2007;132:108S-30S.
- Hansell DM, Bankier AA, MacMahon H, et al. Fleischner Society: glossary of terms for thoracic imaging 1. Radiology 2008;246:697-722. [Crossref] [PubMed]
- Bankier AA, MacMahon H, Goo JM, et al. Recommendations for measuring pulmonary nodules at CT: a statement from the Fleischner Society. Radiology 2017;285:584-600. [Crossref] [PubMed]
- MacMahon H, Naidich DP, Goo JM, et al. Guidelines for management of incidental pulmonary nodules detected on CT images: from the Fleischner Society 2017. Radiology 2017;284:228-43. [Crossref] [PubMed]
- Larici AR, Farchione A, Franchi P, et al. Lung nodules: size still matters. Eur Respir Rev 2017;26:170025. [Crossref] [PubMed]
- Christensen JA, Nathan MA, Mullan BP, et al. Characterization of the solitary pulmonary nodule: 18F-FDG PET versus nodule-enhancement CT. AJR Am J Roentgenol 2006;187:1361-7. [Crossref] [PubMed]
- de Koning HJ, van der Aalst CM, de Jong PA, et al. Reduced lung-cancer mortality with volume CT screening in a randomized trial. N Engl J Med 2020;382:503-13. [Crossref] [PubMed]
- Callister ME, Baldwin DR, Akram AR, et al. British Thoracic Society guidelines for the investigation and management of pulmonary nodules. Thorax 2015;70:ii1-ii54. Erratum in: Thorax 2015;70:1188. [Crossref] [PubMed]
- McWilliams A, Tammemagi MC, Mayo JR, et al. Probability of cancer in pulmonary nodules detected on first screening CT. N Engl J Med 2013;369:910-9. [Crossref] [PubMed]
- Aberle DR, Adams AM, Berg CD, et al. Reduced lung-cancer mortality with low-dose computed tomographic screening. N Engl J Med 2011;365:395-409. [Crossref] [PubMed]
- Horeweg N, van Rosmalen J, Heuvelmans MA, et al. Lung cancer probability in patients with CT-detected pulmonary nodules: a prespecified analysis of data from the NELSON trial of low-dose CT screening. Lancet Oncol 2014;15:1332-41. [Crossref] [PubMed]
- Revel MP, Mannes I, Benzakoun J, et al. Subsolid lung nodule classification: a CT criterion for improving interobserver agreement. Radiology 2018;286:316-25. [Crossref] [PubMed]
- Lee JH, Lim WH, Hong JH, et al. Growth and clinical impact of 6-mm or larger subsolid nodules after 5 years of stability at chest CT. Radiology 2020;295:448-55. [Crossref] [PubMed]
- Naidich DP, Azour L. Managing stable subsolid lung nodules: a possible approach. Radiology 2020;295:456-7. [Crossref] [PubMed]
- Lee JH, Park CM, Lee SM, et al. Persistent pulmonary subsolid nodules with solid portions of 5 mm or smaller: their natural course and predictors of interval growth. Eur Radiol 2016;26:1529-37. [Crossref] [PubMed]
- Cohen JG, Reymond E, Lederlin M, et al. Differentiating pre- and minimally invasive from invasive adenocarcinoma using CT-features in persistent pulmonary part-solid nodules in Caucasian patients. Eur J Radiol 2015;84:738-44. [Crossref] [PubMed]
- van Riel SJ, Sánchez CI, Bankier AA, et al. Observer variability for classification of pulmonary nodules on low-dose CT images and its effect on nodule management. Radiology 2015;277:863-71. [Crossref] [PubMed]
- Truong MT, Ko JP, Rossi SE, et al. Update in the evaluation of the solitary pulmonary nodule. Radiographics 2014;34:1658-79. [Crossref] [PubMed]
- Orlowski HLP, McWilliams S, Mellnick VM, et al. Imaging spectrum of invasive fungal and fungal-like infections. Radiographics 2017;37:1119-34. [Crossref] [PubMed]
- Wang Y, Dong C, Hu Y, et al. Temporal changes of CT findings in 90 patients with COVID-19 pneumonia: a longitudinal study. Radiology 2020;296:E55-64. [Crossref] [PubMed]
- Gaerte SC, Meyer CA, Winer-Muram HT, et al. Fat-containing lesions of the chest. Radiographics 2002;22:S61-78. [Crossref] [PubMed]
- Woodring JH, Fried AM. Significance of wall thickness in solitary cavities of the lung: a follow-up study. AJR Am J Roentgenol 1983;140:473-4. [Crossref] [PubMed]
- Chou SHS, Kicska G, Kanne JP, et al. Cheerio sign. J Thorac Imaging 2013;28:W4. [Crossref] [PubMed]
- Trotman-Dickenson B. Cystic lung disease: achieving a radiologic diagnosis. Eur J Radiol 2014;83:39-46. [Crossref] [PubMed]
- Gardiner N, Jogai S, Wallis A. The revised lung adenocarcinoma classification-an imaging guide. J Thorac Dis 2014;6:S537-46. [PubMed]
- Lindell RM, Hartman TE, Swensen SJ, et al. Five-year lung cancer screening experience: CT appearance, growth rate, location, and histologic features of 61 lung cancers. Radiology 2007;242:555-62. [Crossref] [PubMed]
- de Hoop B, van Ginneken B, Gietema H, et al. Pulmonary perifissural nodules on CT scans: rapid growth is not a predictor of malignancy. Radiology 2012;265:611-6. [Crossref] [PubMed]
- Schreuder A, van Ginneken B, Scholten ET, et al. Classification of CT pulmonary opacities as perifissural nodules: reader variability. Radiology 2018;288:867-75. [Crossref] [PubMed]
- Wilson DO, Weissfeld JL, Balkan A, et al. Association of radiographic emphysema and airflow obstruction with lung cancer. Am J Respir Crit Care Med 2008;178:738-44. [Crossref] [PubMed]
- de Torres JP, Bastarrika G, Wisnivesky JP, et al. Assessing the relationship between lung cancer risk and emphysema detected on low-dose CT of the chest. Chest 2007;132:1932-8. [Crossref] [PubMed]
- Kwak N, Park CM, Lee J, et al. Lung cancer risk among patients with combined pulmonary fibrosis and emphysema. Respir Med 2014;108:524-30. [Crossref] [PubMed]
- Yoshida R, Arakawa H, Kaji Y. Lung cancer in chronic interstitial pneumonia: early manifestation from serial CT observations. AJR Am J Roentgenol 2012;199:85-90. [Crossref] [PubMed]
- Fletcher JW, Kymes SM, Gould M, et al. A comparison of the diagnostic accuracy of 18F-FDG PET and CT in the characterization of solitary pulmonary nodules. J Nucl Med 2008;49:179-85. [Crossref] [PubMed]
- Gould MK, Maclean CC, Kuschner WG, et al. Accuracy of positron emission tomography for diagnosis of pulmonary nodules and mass lesions: a meta-analysis. JAMA 2001;285:914-24. [Crossref] [PubMed]
- Herder GJ, van Tinteren H, Golding RP, et al. Clinical prediction model to characterize pulmonary nodules: validation and added value of 18F-fluorodeoxyglucose positron emission tomography. Chest 2005;128:2490-6. [Crossref] [PubMed]
- Al-Ameri A, Malhotra P, Thygesen H, et al. Risk of malignancy in pulmonary nodules: a validation study of four prediction models. Lung Cancer 2015;89:27-30. [Crossref] [PubMed]
- Teoh EJ, McGowan DR, Bradley KM, et al. Novel penalised likelihood reconstruction of PET in the assessment of histologically verified small pulmonary nodules. Eur Radiol 2016;26:576-84. [Crossref] [PubMed]
- Murphy DJ, Royle L, Chalampalakis Z, et al. The effect of a novel Bayesian penalised likelihood PET reconstruction algorithm on the assessment of malignancy risk in solitary pulmonary nodules according to the British Thoracic Society guidelines. Eur J Radiol 2019;117:149-55. [Crossref] [PubMed]
- Nomori H, Watanabe K, Ohtsuka T, et al. Evaluation of F-18 fluorodeoxyglucose (FDG) PET scanning for pulmonary nodules less than 3 cm in diameter, with special reference to the CT images. Lung Cancer 2004;45:19-27. [Crossref] [PubMed]
- Hofman MS, Lau WFE, Hicks RJ. Somatostatin receptor imaging with 68Ga DOTATATE PET/CT: clinical utility, normal patterns, pearls, and pitfalls in interpretation. Radiographics 2015;35:500-16. [Crossref] [PubMed]
- Wu LM, Xu JR, Hua J, et al. Can diffusion-weighted imaging be used as a reliable sequence in the detection of malignant pulmonary nodules and masses? Magn Reson Imaging 2013;31:235-46. [Crossref] [PubMed]
- Mori T, Nomori H, Ikeda K, et al. Diffusion-weighted magnetic resonance imaging for diagnosing malignant pulmonary nodules/masses: comparison with positron emission tomography. J Thorac Oncol 2008;3:358-64. [Crossref] [PubMed]
- Schaefer JF, Vollmar J, Schick F, et al. Solitary pulmonary nodules: dynamic contrast-enhanced MR imaging--perfusion differences in malignant and benign lesions. Radiology 2004;232:544-53. [Crossref] [PubMed]
- Nair A, Devaraj A, Callister MEJ, et al. The Fleischner Society 2017 and British Thoracic Society 2015 guidelines for managing pulmonary nodules: keep calm and carry on. Thorax 2018;73:806-12. [Crossref]
- Devaraj A, van Ginneken B, Nair A, et al. Use of volumetry for lung nodule management: theory and practice. Radiology 2017;284:630-44. [Crossref] [PubMed]
- Lee HY, Choi YL, Lee KS, et al. Pure ground-glass opacity neoplastic lung nodules: histopathology, imaging, and management. AJR Am J Roentgenol 2014;202:W224-33. [Crossref] [PubMed]
- Lassen BC, Jacobs C, Kuhnigk JM, et al. Robust semi-automatic segmentation of pulmonary subsolid nodules in chest computed tomography scans. Phys Med Biol 2015;60:1307-23. [Crossref] [PubMed]
- Gambhir SS, Shepherd JE, Shah BD, et al. Analytical decision model for the cost-effective management of solitary pulmonary nodules. J Clin Oncol 1998;16:2113-25. [Crossref] [PubMed]
- Lejeune C, Zahouri Al K, Woronoff-Lemsi MC, et al. Use of a decision analysis model to assess the medicoeconomic implications of FDG PET imaging in diagnosing a solitary pulmonary nodule. Eur J Health Econ 2005;6:203-14. [Crossref] [PubMed]
- Swensen SJ, Silverstein MD, Ilstrup DM, et al. The probability of malignancy in solitary pulmonary nodules. Application to small radiologically indeterminate nodules. Arch Intern Med 1997;157:849-55. [Crossref] [PubMed]
- Hammer MM, Nachiappan AC, Barbosa EJM. Limited utility of pulmonary nodule risk calculators for managing large nodules. Curr Probl Diagn Radiol 2018;47:23-7. [Crossref] [PubMed]
- Girvin F, Ko JP. Pulmonary nodules: detection, assessment, and CAD. AJR Am J Roentgenol 2008;191:1057-69. [Crossref] [PubMed]
- Murphy DJ, Bille A. Using a radiomics-derived classifier to distinguish between lung adenocarcinomas and granulomas—where are we now? J Med Artif Intell 2018;1:10. [Crossref]
- Dennie C, Thornhill R, Sethi-Virmani V, et al. Role of quantitative computed tomography texture analysis in the differentiation of primary lung cancer and granulomatous nodules. Quant Imaging Med Surg 2016;6:6-15. [PubMed]
- Kido S, Kuriyama K, Higashiyama M, et al. Fractal analysis of internal and peripheral textures of small peripheral bronchogenic carcinomas in thin-section computed tomography: comparison of bronchioloalveolar cell carcinomas with nonbronchioloalveolar cell carcinomas. J Comput Assist Tomogr 2003;27:56-61. [Crossref] [PubMed]
- Orooji M, Alilou M, Rakshit S, et al. Combination of computer extracted shape and texture features enables discrimination of granulomas from adenocarcinoma on chest computed tomography. J Med Imaging (Bellingham) 2018;5:024501. [Crossref] [PubMed]
- Son JY, Lee HY, Lee KS, et al. Quantitative CT analysis of pulmonary ground-glass opacity nodules for the distinction of invasive adenocarcinoma from pre-invasive or minimally invasive adenocarcinoma. PLoS One 2014;9:e104066. [Crossref] [PubMed]
- Ikeda K, Awai K, Mori T, et al. Differential diagnosis of ground-glass opacity nodules: CT number analysis by three-dimensional computerized quantification. Chest 2007;132:984-90. [Crossref] [PubMed]
- Ganeshan B, Panayiotou E, Burnand K, et al. Tumour heterogeneity in non-small cell lung carcinoma assessed by CT texture analysis: a potential marker of survival. Eur Radiol 2012;22:796-802. [Crossref] [PubMed]
- Li M, Narayan V, Gill RR, et al. Computer-aided diagnosis of ground-glass opacity nodules using open-source software for quantifying tumor heterogeneity. AJR Am J Roentgenol 2017;209:1216-27. [Crossref] [PubMed]
- de Margerie-Mellon C, Gill RR, Salazar P, et al. Assessing invasiveness of subsolid lung adenocarcinomas with combined attenuation and geometric feature models. Sci Rep 2020;10:14585. [Crossref] [PubMed]
- Cui S, Ming S, Lin Y, et al. Development and clinical application of deep learning model for lung nodules screening on CT images. Sci Rep 2020;10:13657. [Crossref] [PubMed]
- Ardila D, Kiraly AP, Bharadwaj S, et al. End-to-end lung cancer screening with three-dimensional deep learning on low-dose chest computed tomography. Nat Med 2019;25:954-61. [Crossref] [PubMed]
- Huang P, Lin CT, Li Y, et al. Prediction of lung cancer risk at follow-up screening with low-dose CT: a training and validation study of a deep learning method. Lancet Digit Health 2019;1:e353-62. [Crossref] [PubMed]
Cite this article as: Murphy DJ, Gill RR. Radiographic assessment of small lung nodules: what can we do and what information does it give us? Curr Chall Thorac Surg 2022;4:32.