Hierarchal clustering for the identification of distinct rib fracture patterns
Highlight box
Key findings
• Rib fracture clustering analysis demonstrated important clinical outcome associations in motor vehicle and motorcycle accident injured patients.
What is known and what is new?
• Rib fractures and chest wall injuries are common and often described in very broad imprecise categorical terminology.
• Modern fracture taxonomy increases the descriptive precision of injuries, but analyses of the new possible fracture subsets is hindered by difficulty parsing the density of injury data.
What is the implication, and what should change now?
• We present a novel analytic technique to assist in rib fracture pattern recognition and demonstrate its use in the identification of clinically relevant outcome associations.
Introduction
Background
Bony thoracic injury is one of the most commonly encountered injuries and a major cause of post injury morbidity (1,2). The incidence of rib fracture evaluations in the US is increasing and was as high as 944,000 (±134,000) emergency room visits in 2020 alone, up from 303,000 in 2000 (3). The consequences of rib fractures are well-known and vary widely from severe pain, to hospital readmission, to infectious sequelae, to long term disability (2,4-7). Despite the increased interest in rib injury, rib fracture study remains limited by the ability to process only partial descriptors of injury. Examples of such limitations include comparing the presence or absence of fractures, numbers of fractures grouped into categories, presence or absence of bilaterality, segmentality, flail, displacement or some combination therein.
Rationale and knowledge gap
These limitations prevent clinicians and researchers of chest injury from graduating injuries further, and may impute assumptions into the existing body of literature. For example, if a patient sustains a chest injury with an abbreviated injury scale (AIS) of 3, they can have at least 3 rib fractures in any permutation. Under traditional clinical methods of study, this could mean that fractures on the posterior aspects of ribs 1, 2, and 12 (which are stabilized by the transverse process and erector musculature) are equivalent to bicorticately displaced anterolateral 4, 5, and 6 fractures (which are more mobile during the respiratory cycle). Similarly, there would be no differentiation from a pattern where every other rib is fractured (such as 3rd, 5th, and 7th ribs fractured) and a stable unbroken rib is found between all levels of fractures. Newer data sets with more anatomically dense information are being constructed following the recent update to chest injury taxonomy (8) but increased data density presents an analytic challenge.
These new data sets allow routine cross-sectional imaging studies to be used to describe rib fractures in their cranial-caudal position by rib number, spatial location from anterior to posterior, as well as classify degree of displacement. This additional information can produce highly granular chest injury maps (9). Traditional analytic approaches to these maps are problematic when considering that six rib fractures across such a rib grid could produce as many as 3.6 billion fracture pattern permutations. Comparisons using Chi squared, Fischer’s tests, or regressions analysis, for example, with data sets of this density are laborious and may ignore potentially significant spatial relationships. Furthermore, it may be impossible to accrue enough permutations to satisfy significance with traditional methods.
Objective
Methods to handle large volume spatially significant data are available. Hierarchical clustering has been used in the analysis of large data sets such gene expression patterns in high-throughput genetics, in addition to detecting patterns in healthcare utilization, video game optimization, and retail spending patterns (9-12). Hierarchical clustering aids in the identification of patterns through the organization of ordinal variables such as rib fracture locations. By using the coordinate representation of anatomic injury (such as a rib fracture grid matrix) it may be possible to apply hierarchical clustering analysis to rib fractures. We hypothesize that hierarchical clustering could be used to detect clinically important associations between locations of rib fractures, while maintaining fidelity to the geographic loci of each rib, employing rib number taxonomy and anterior-posterior location of the rib fracture. Specifically, we tested: can such analysis demonstrate differences in fracture patterns between patients seen following motor vehicle collisions (MVCs) and motorcycle collisions (MCCs), and can pattern differences be demonstrated between patients who do and do not require tracheostomy? We present this article in accordance with the STROBE reporting checklist (available at https://ccts.amegroups.com/article/view/10.21037ccts-23-17/rc).
Methods
Clinical data collection
To test the hypothesis, a large data set inclusive of detailed loci of anatomic injury was needed. The Chest Wall Injury Society (CWIS) maintains a large database of operative and non-operative bony thoracic injuries with clinical correlates known as the Chest Injury International Database (CIID). The CIID was reviewed for patients presenting with multiple rib fractures following injury including information about injury mechanism and hospital events including tracheostomy. The study was conducted in accordance with the Declaration of Helsinki (as revised in 2013). The study was approved by the institutional review board of the University of Cincinnati (No.: 2020-0330) and individual consent for this retrospective analysis was waived. Rib fractures are described as per current taxonomy by cranial to caudal rib number, one of five positions on the rib from anterior to posterior, and degree of displacement (8). A composite five by twelve matrix of each hemithorax was created to describe corresponding fracture site frequency in each geographic location. Hemithorax matrices were combined into a 10×12 matrix to account for proximity of bilateral positions, bound at the midline anteriorly. Sternal fractures were not included in the composite matrix due to inadequate detail of sternal fracture location. Dichotomous comparative groups for each rib grid analysis were created: MCCs versus MVCs, and patients requiring tracheostomy versus patients who did not.
Statistical analysis
Data from comparator groups were collected and organized into paired conditions with non-overlapping cohorts. Fracture frequency matrices were analyzed using R packages ‘ComplexHeatMap’, ‘mclust’, ‘hclust’ and ‘dendextend’ (R studio—Cary, NC, USA) (9,13-15). Heat-maps were generated using an assigned color gradient corresponding clustering patterns of rib fracture frequencies. Rib fracture frequencies were sorted by hierarchical clustering with Euclidean distancing to yield dendrograms of highly associated rib fractures. Bayesian information criterion (BIC) was utilized to determine the optimal number of clusters with the pattern, using the ‘mclust’ package (13). Distancing was calibrated with multiple clustering algorithms and the best-fitting model was selected. After optimal clusters were determined, dendrograms were isolated for clinical variables. Descriptive statistics for dendrogram similarity were used, as well as powered statistical tests to measure degree of correlative branching between two dendrograms (14,15). All powered analysis employed a significance level of a P value of less than 0.05.
For confirmatory study, only one axis variable could be compared at a time. Entanglement co-efficients were used to describe degree of clustering similarity and dendrogram alignment (14) and powered statistical analysis was performed via the Baker’s gamma index (BGI). Additionally, binomial regression analysis for outcome variable of tracheostomy was performed on the 325 patients’ individual fracture grids to examine accuracy of fracture frequency clustering.
Results
A total of 613 patients who had blunt chest injury following either MVC or MCC were reviewed from an international multi-institutional database. Of these 443 patients with 3,732 rib fractures presented after MVC (average 8.3 fractures) and 170 patients with 1,412 rib fractures presented following MCC (average 8.4 fractures). To account for incomplete data, a single institutional subset of the database was evaluated for tracheostomy and 325 patients were identified for analysis. Of these 43 patients with 570 fractures underwent tracheostomy (average 13.3 fractures) and 282 patients with 2,859 fractures did not (average 10.1 fractures). Demographic and selected treatment information can be seen in Tables 1,2.
Table 1
Characteristics | Motorcycle collision (n=170) | Motor vehicle collision (n=443) |
---|---|---|
Age (years), median (IQR) | 46.5 (36, 62) | 51 (37, 65) |
Male, n [%] | 150 [88] | 251 [57] |
ISS, median (IQR) | 24 (17, 34) | 25 (18, 36) |
Fractures, n | 1,412 | 3,732 |
Fracture per patient, n | 8.4 | 8.3 |
SSRF, n [%] | 27 [16] | 82 [19] |
Optimal branched clusters, n | 9 | 9 |
Cluster distancing method with best fit | EEI | EEI |
Fracture locations in highest frequency cluster | ||
Rib numbers | 4, 5, 6 | 4, 5, 6, 7 |
Rib AP positions | Lateral (right, left), posterior (right, left), posterior-lateral (left) | Lateral (right, left) |
Entanglement co-efficient (AP positions) | 0.53 | |
Entanglement co-efficient left side (rib number) | 0.77 | |
Entanglement co-efficient right side (rib number) | 0.75 | |
BGI P value for similarity (AP positions) | 0.19 | |
BGI P value for similarity right side (rib number) | <0.01 | |
BGI P value for similarity left side (rib number) | 0.03 |
IQR, interquartile range; ISS, injury severity score; SSRF, surgical stabilization of rib fractures; EEI, ellipsoidal, equal volume and shape mClust; AP, anterior-posterior; BGI, Baker’s gamma index.
Table 2
Characteristics | Tracheostomy (n=43) | No tracheostomy (n=282) |
---|---|---|
Age (years), median (IQR) | 54.5 (35, 68) | 55 (42, 67) |
Male, n [%] | 34 [79] | 180 [64] |
ISS, median (IQR) | 36 (34, 43) | 20 (14, 29) |
Fractures, n | 570 | 2,859 |
Average fracture per patient, n | 13.3 | 10.1 |
SSRF, n [%] | 14 [33] | 73 [26] |
Optimal branched clusters, n | 8 | 8 |
Cluster distancing method with best fit | EEI | EEI |
Fracture locations in highest frequency cluster | ||
Rib numbers | 3, 4, 5, 6, 7, 8 | 4, 5, 6, 7, 8 |
Rib AP positions | Posterior-lateral (right, left) | Lateral (right, left) |
Entanglement co-efficient (AP positions) | 0.46 | |
Entanglement co-efficient left side (rib number) | 0.94 | |
Entanglement co-efficient right side (rib number) | 0.91 | |
BGI P value for similarity (AP positions) | 0.21 | |
BGI P value for similarity right side (rib number) | <0.01 | |
BGI P value for similarity left side (rib number) | <0.01 |
IQR, interquartile range; ISS, injury severity score; SSRF, surgical stabilization of rib fractures; EEI, ellipsoidal, equal volume and shape mClust; AP, anterior-posterior; BGI, Baker’s gamma index.
Fracture location frequencies sustained in MVCs were compared to those in MCCs, and clustering analysis performed. Results of clustering are shown in heat maps with color gradients depicting the scaled proportion of fractures by coordinate location, illustrating high and low frequencies (Figure 1). The optimal number of clusters as determined by BIC was 9 in elliptical distancing model EEI (ellipsoidal, equal volume and shape mClust) for both MVC and MCC. The most closely associated clusters (left upper corner) are diagrammatically displayed in approximate chest wall location to assist in pattern visualization. For the MVC group, the highest frequency cluster included bilateral ribs 4, 5, 6, and 7 laterally. For the MCC group, the highest frequency clusters included bilateral ribs 4, 5, and 6 laterally and posteriorly, as well as left posterior-lateral 4, 5, and 6 rib fractures (Table 1). Entanglement for the branched dendrograms between MVC and MCC were compared along the anterior to posterior axis (co-efficient 0.53) and by cranial to caudal rib number on either side (co-efficients 0.75 right and 0.77 left) (Table 1, Figure S1). Similarity of fracture pattern location between MVC and MCC was evaluated using BGI analysis and demonstrated similar clustering by rib number (right: P<0.01 and left: P=0.03) but not by anterior-posterior location (P=0.19) (Table 1, Figure S2).
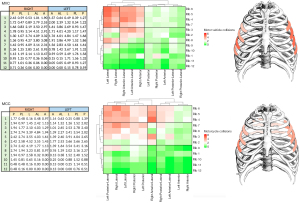
Similarly, the populations of tracheostomy and no tracheostomy were analyzed for patterns. The optimal number of clusters by BIC was determined to be 8 using the same model for both patients receiving tracheostomy and not. For the tracheostomy group, the highest frequency cluster included bilateral ribs 3, 4, 5, 6, 7, and 8 posterior-laterally.
For the no tracheostomy group, the highest frequency clusters included bilateral ribs 4, 5, 6, 7, and 8 laterally (Table 2). No significant similarities between tracheostomy and no tracheostomy were identified along anterior-posterior axis (entanglement co-efficient 0.46). However cranial to caudal positioning demonstrated similarities in a grouping of ribs 1, 9, 10, 11, and 12, and a second group of ribs 7 and 8 (entanglement co-efficient 0.94 and 0.91 for the left and right sides respectively) (Table 2, Figure S3). Similarity of fracture pattern location between tracheostomy and no tracheostomy using BGI analysis demonstrated similar clustering by rib number (P<0.01) but not by anterior-posterior location (P=0.21) (Table 1, Figure S4).
Tracheostomy cluster results were validated by binomial regression analysis on each of 325 patients’ individual fracture grids (Table 3). Seventeen location variables based on dendrogram objects (12 ribs and five anterior-posterior locations) were examined. Location incidence per patient, standard deviation and confidence intervals are listed in Table 4. The posterior-lateral position was significantly associated with receipt of tracheostomy and remained significant when controlled for other anterior-posterior positions in the regression model (P<0.01) (Table 5). Rib 3 fractures had differential clustering locations between patients with tracheostomy and those without. Rib 3 was included in the highest frequency cluster for tracheostomy, but not in the highest frequency fracture cluster for patients without tracheostomies (Table 5, Figure 2). In the regression model, rib 3 was significantly associated with an increased risk for tracheostomy when controlled for other rib numbers (P=0.04) (Table 5). All rib fracture clustering analyses are available at https://cdn.amegroups.cn/static/public/ccts-23-17-1.pdf.
Table 3
Patients | Fractures | |
---|---|---|
Tracheostomy | 43 | 570 |
No tracheostomy | 282 | 2,859 |
Table 4
Mean | Standard deviation | 95% CI | Range | |
---|---|---|---|---|
Fractures in anatomic location | ||||
Anterior | 1.039 | 1.807 | 0.843, 1.236 | (0, 9) |
Anterolateral | 2.403 | 3.062 | 2.071, 2.736 | (0, 17) |
Lateral | 3.229 | 2.886 | 2.915, 3.543 | (0,18) |
Posterior-lateral | 2.122 | 2.963 | 1.799, 2.445 | (0, 18) |
Posterior | 1.477 | 2.506 | 1.204, 1.749 | (0, 12) |
Rib number | ||||
Rib 1 | 0.36 | 0.686 | 0.286, 0.435 | (0, 4) |
Rib 2 | 0.685 | 0.894 | 0.588, 0.782 | (0, 4) |
Rib 3 | 1.064 | 1.009 | 0.954, 1.173 | (0, 5) |
Rib 4 | 1.266 | 0.987 | 1.158, 1.373 | (0, 4) |
Rib 5 | 1.416 | 0.958 | 1.311, 1.520 | (0, 5) |
Rib 6 | 1.413 | 0.942 | 1.310, 1.515 | (0, 6) |
Rib 7 | 1.342 | 0.899 | 1.245, 1.440 | (0, 5) |
Rib 8 | 1.067 | 0.848 | 0.975, 1.159 | (0, 5) |
Rib 9 | 0.779 | 0.825 | 0.690, 0.869 | (0, 4) |
Rib 10 | 0.502 | 0.704 | 0.425, 0.578 | (0, 3) |
Rib 11 | 0.266 | 0.542 | 0.207, 0.325 | (0, 3) |
Rib 12 | 0.119 | 0.343 | 0.082, 0.157 | (0, 2) |
CI, confidence interval.
Table 5
Estimate | Standard error | Z value | P value | |
---|---|---|---|---|
Anterior-posterior position regression | ||||
Intercept | −2.638 | 0.368 | −7.164 | <0.01 |
Anterior | 0.185 | 0.084 | 2.204 | 0.03 |
Anterolateral | 0.040 | 0.055 | 0.723 | 0.47 |
Lateral | −0.129 | 0.067 | −1.919 | 0.06 |
Posterior-lateral | 0.172 | 0.051 | 3.389 | <0.01 |
Posterior | 0.195 | 0.061 | 3.190 | <0.01 |
Rib number regression | ||||
Intercept | −2.707 | 0.376 | −7.200 | <0.01 |
Rib 1 | 0.272 | 0.254 | 1.073 | 0.28 |
Rib 2 | −0.002 | 0.283 | −0.008 | 0.99 |
Rib 3 | 0.663 | 0.326 | 2.033 | 0.04 |
Rib 4 | 0.259 | 0.349 | −0.741 | 0.46 |
Rib 5 | −0.153 | 0.342 | −0.447 | 0.66 |
Rib 6 | 0.135 | 0.304 | 0.442 | 0.66 |
Rib 7 | 0.238 | 0.284 | 0.835 | 0.40 |
Rib 8 | −0.724 | 0.354 | −2.047 | 0.04 |
Rib 9 | 0.679 | 0.330 | 2.057 | 0.04 |
Rib 10 | −0.158 | 0.387 | −0.408 | 0.68 |
Rib 11 | 0.054 | 0.441 | 0.122 | 0.90 |
Rib 12 | 1.156 | 0.512 | 2.260 | 0.02 |
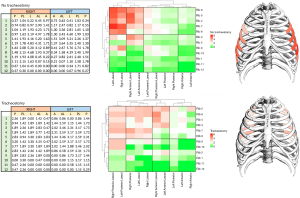
Discussion
Key findings
With the expanded availability of large volume detailed data sets, the opportunity to characterize rib fractures in greater spatial detail is increasingly available. We report on the use of a high-volume data analytic technique to identify and characterize rib injury patterns with clinical correlates. Using this technique, we are able to demonstrate that differential rib injury patterns can be associated with mechanistic variables such as MVCs and MCCs, and clinical outcomes such as need for tracheostomy. Specifically, we are able to show that posterior-lateral patterns of injury with a focused around rib 3, appear to have a high association with tracheostomy.
Explanation of findings
Interpretation of these findings needs to be carefully considered. In the MVC and MCC example, one interpretation could be that motorcycle injuries demonstrate a posterior rib fracture predominance because it includes 50% of the possible anterior to posterior positions across the three highest incidence ribs. This is contrasted against the MVC fracture distribution which is much more condensed along lateral positions across four ribs. Using BGI analysis, the pattern differences between MVC and MCC are confirmed only to be unique in the anterior-posterior axis. With this information, one can speculate that differences may be explained by the directional force and energy dispersion vectors experienced by the automobile passenger (oriented along cardinal directions) as opposed to the cyclist (randomly or radially oriented forces from tumbling). Similar observations have been made in fractures resulting from CPR and frontal-impact auto collisions (16). However, in clinical application more conservative contextualization of an identified pattern is necessary to prevent extrapolation of results.
Likewise, the apparent finding in the cohort evaluated for tracheostomy appears to highlight posterior and posterior-lateral fractures. The overall fracture pattern demonstrated on the clustered heat-map (Figure 2) for tracheostomy patients appears to be diffuse, whereas the non-tracheostomy patients appear to cluster laterally. Curiously, differential fracture clustering of rib 3 was also seen in patients who received tracheostomy. Using binomial regression analysis as a more traditional analytic approach in large multi-axis data sets is laborious (up to 34 anatomic variables); however, this did allow confirmation of both the anterior-posterior and rib number as predictors of tracheostomy. The interpretation of such findings is not clear from this analysis. It must be emphasized that clustering analysis alone cannot produce definitive conclusions about isolated significant associations between fracture location and clinical feature. As a tool, this technique may be important as a hypothesis generator (for example: “Is the pattern of anterior and posterior-lateral fractures in the trach population indicative of ineffective analgesia?”) that can propel further investigation with scalability not available in traditional regression analysis. This technique may allow connecting other observations, such as the associations of rib fracture pain, pulmonary contusions and poor airway protection seen in the setting of tracheostomy (17-19), with the early identification of an injury pattern.
Comparisons with similar researches
The techniques used here are novel applications of known technologies. For example, rudimentary fracture location heat map analysis is emerging (20) in response to the aforementioned shortcomings of standard rib fracture analysis. The expanded data density of single patient or population level rib fracture incidences necessitates leveraging methods outside traditional biomedical statistics. Hierarchical clustering has been utilized in a variety of fields, but most notably in the analysis of genetic expression where datasets include tens of thousands of variables needing logical sorting (9-12). The cross-disciplinary use of hierarchical clustering is at its core a process of categorizing ordinal variables into patterns. The translation of clustering methodology in application to rib fractures is necessary to begin to explore the newly available loci data for relevant pattern detection. For example, knowing that six rib fractures is a cut off for mortality (21) does not differentiate between the 3.6 billion fracture pattern combinations of having six fractures according to contemporary location description. The use of Euclidean distancing offers the hope of being able to identify clinically relevant clustering of fractures and patterns without needing to create a data set large enough to detect “non-Euclidean” significance.
Limitations
Our study is not without its limitations. First, the data used for testing is not controlled or representative of a complete consecutive set. However, this limitation is mitigated by assaying a historically uncontrolled and deep intersecting set of anatomic measures for which biases and confounders have not yet been identified. Second, the clustering methodology was dependent upon several optimizations and choices. For example, the BIC output for optimal cluster determination is an integer value and relies on the manual orientation of the number of clusters along the axes. While the differences between clusters are often easily delineated from the corresponding dendrogram, such as in this sample, there may be instances where the clusters are less obvious. In other disciplines, when clusters are subjectively unclear, it is widely accepted for investigators to draw the lines along the most biologically relevant clusters, which may produce observer bias. Another optimization included choosing ‘EEI’ horizontally oriented elliptical distancing. ‘EEI’ produced the best fit in these comparisons but may not capture some cranial-caudal oriented patterns with as much sensitivity as other distancing methods. Third, this application is limited by its analysis of aggregated frequencies of rib fracture data, and therefore may not be sensitive to infrequent, but highly impactful, patterns of rib fracture. This last limitation is anticipated to be mitigated over time by applying this method to larger data sets. Finally, utilization of this technique is currently limited by access to data sets that have detailed fracture location information. With current methods, these sets are labor intensive to produce, and generally speaking small compared to decades old injury databases. Future work will be dependent upon developing the ability to scale anatomic, physiologic, and clinical narrative data rich sets.
Implications and actions needed
Hierarchical clustering is a method for detecting patterns of association between ordinal variables in highly granular data sets where traditional methods are not feasible. In this two-axis application of clustering, a combination clustering process sorted rib fracture vectors of rib number and anterior to posterior position into patterns that appear to correlate with clinically relevant events. Further, clustering ordered fractures into configurations that described clinically relevant associations between groups of patients by injury mechanism and outcome. Similarities between regression model and clustering output reinforced the utility of rib fracture clustering for global organization and phenotype detection in injured patients. In processing vast datasets, it may be more time-effective to globally organize data first to aid in identification of patterns, phenotypes, and variables associated with experimental variables. This process represents a tool that has the potential to address the next frontier of deep data, and ever more nuanced rib fracture location and descriptive detail. The application of this process for rib injury may be limited only by the structuring of clinical questions for novel investigation.
Conclusions
We are able to demonstrate that dense anatomic data with rib fracture location can reveal patterns unique to both injury mechanism and clinical outcome. Using high-level clustering, a pattern of more posteriorly located rib fracture is significantly more frequently in seen in motorcycle injuries compared to motor-vehicle collisions. Moreover, posterior-lateral fracture patterns centered around rib 3 appear to significantly associate with need for tracheostomy. Hierarchical clustering appears to be useful in sorting large datasets and can serve as a preprocessing step for data analysis, to identify patterns and phenotypes and aid developing clinically oriented hypotheses.
Acknowledgments
The authors would like to thank SaraAnn Whitbeck and Jonathan Jesneck, as well as the Chest Wall Injury Society for their assistance with construction and maintenance of the Chest Injury International Database. This research was presented to the Chest Wall Injury Society, April 2023, Charlotte, NC, USA.
Funding: None.
Footnote
Reporting Checklist: The authors have completed the STROBE reporting checklist. Available at https://ccts.amegroups.com/article/view/10.21037/ccts-23-17/rc
Data Sharing Statement: Available at https://ccts.amegroups.com/article/view/10.21037/ccts-23-17/dss
Peer Review File: Available at https://ccts.amegroups.com/article/view/10.21037/ccts-23-17/prf
Conflicts of Interest: All authors have completed the ICMJE uniform disclosure form (available at https://ccts.amegroups.com/article/view/10.21037/ccts-23-17/coif). The authors have no conflicts of interest to declare.
Ethical Statement: The authors are accountable for all aspects of the work in ensuring that questions related to the accuracy or integrity of any part of the work are appropriately investigated and resolved. The study was conducted in accordance with the Declaration of Helsinki (as revised in 2013). The study was approved by the institutional review board of the University of Cincinnati (N
Open Access Statement: This is an Open Access article distributed in accordance with the Creative Commons Attribution-NonCommercial-NoDerivs 4.0 International License (CC BY-NC-ND 4.0), which permits the non-commercial replication and distribution of the article with the strict proviso that no changes or edits are made and the original work is properly cited (including links to both the formal publication through the relevant DOI and the license). See: https://creativecommons.org/licenses/by-nc-nd/4.0/.
References
- Kasotakis G, Hasenboehler EA, Streib EW, et al. Operative fixation of rib fractures after blunt trauma: A practice management guideline from the Eastern Association for the Surgery of Trauma. J Trauma Acute Care Surg 2017;82:618-26. [Crossref] [PubMed]
- Marasco S, Liew S, Edwards E, et al. Analysis of bone healing in flail chest injury: do we need to fix both fractures per rib? J Trauma Acute Care Surg 2014;77:452-8. [Crossref] [PubMed]
- Marasco SF, Balogh ZJ, Wullschleger ME, et al. Rib fixation in non-ventilator-dependent chest wall injuries: A prospective randomized trial. J Trauma Acute Care Surg 2022;92:1047-53. [Crossref] [PubMed]
- Craxford S, Owyang D, Marson B, et al. Surgical management of rib fractures after blunt trauma: a systematic review and meta-analysis of randomised controlled trials. Ann R Coll Surg Engl 2022;104:249-56. [PubMed]
- Dehghan N, Nauth A, Schemitsch E, et al. Operative vs Nonoperative Treatment of Acute Unstable Chest Wall Injuries: A Randomized Clinical Trial. JAMA Surg 2022;157:983-90. [Crossref] [PubMed]
- Pieracci FM, Agarwal S, Doben A, et al. Indications for surgical stabilization of rib fractures in patients without flail chest: surveyed opinions of members of the Chest Wall Injury Society. Int Orthop 2018;42:401-8. [Crossref] [PubMed]
- Pieracci FM, Leasia K, Bauman Z, et al. A multicenter, prospective, controlled clinical trial of surgical stabilization of rib fractures in patients with severe, nonflail fracture patterns (Chest Wall Injury Society NONFLAIL). J Trauma Acute Care Surg 2020;88:249-57. [Crossref] [PubMed]
- Edwards JG, Clarke P, Pieracci FM, et al. Taxonomy of multiple rib fractures: Results of the chest wall injury society international consensus survey. J Trauma Acute Care Surg 2020;88:e40-5. [Crossref] [PubMed]
- Gu Z, Eils R, Schlesner M. Complex heatmaps reveal patterns and correlations in multidimensional genomic data. Bioinformatics 2016;32:2847-9. [Crossref] [PubMed]
- Chen IF, Lu CJ. Sales forecasting by combining clustering and machine-learning techniques for computer retailing. Neural Computing and Applications 2017;28:2633-47. [Crossref]
- Drachen A, Sifa R, Bauckhage C, et al. Guns, swords and data: Clustering of player behavior in computer games in the wild. 2012 IEEE Conference on Computational Intelligence and Games (CIG). Granada: IEEE; 2012.
- Leech RM, McNaughton SA, Timperio A. The clustering of diet, physical activity and sedentary behavior in children and adolescents: a review. Int J Behav Nutr Phys Act 2014;11:4. [Crossref] [PubMed]
- Scrucca L, Fop M, Murphy TB, et al. mclust 5: Clustering, Classification and Density Estimation Using Gaussian Finite Mixture Models. R J 2016;8:289-317. [Crossref] [PubMed]
- Galili T. dendextend: an R package for visualizing, adjusting and comparing trees of hierarchical clustering. Bioinformatics 2015;31:3718-20. [Crossref] [PubMed]
- Lapointe FJ, Legendre P. Comparison tests for dendrograms: A comparative evaluation. Journal of Classification 1995;12:265-82. [Crossref]
- Liebsch C, Seiffert T, Vlcek M, et al. Patterns of serial rib fractures after blunt chest trauma: An analysis of 380 cases. PLoS One 2019;14:e0224105. [Crossref] [PubMed]
- Tyburski JG, Collinge JD, Wilson RF, et al. Pulmonary contusions: quantifying the lesions on chest X-ray films and the factors affecting prognosis. J Trauma 1999;46:833-8. [Crossref] [PubMed]
- Chapman BC, Herbert B, Rodil M, et al. RibScore: A novel radiographic score based on fracture pattern that predicts pneumonia, respiratory failure, and tracheostomy. J Trauma Acute Care Surg 2016;80:95-101. [Crossref] [PubMed]
- Zhang B, Li GK, Wang YR, et al. Prediction of factors influencing the timing and prognosis of early tracheostomy in patients with multiple rib fractures: A propensity score matching analysis. Front Surg 2022;9:944971. [Crossref] [PubMed]
- Thomas CN, Lindquist TJ, Paull TZ, et al. Mapping of common rib fracture patterns and the subscapular flail chest associated with operative scapula fractures. J Trauma Acute Care Surg 2021;91:940-6. [Crossref] [PubMed]
- Flagel BT, Luchette FA, Reed RL, et al. Half-a-dozen ribs: the breakpoint for mortality. Surgery 2005;138:717-23; discussion 723-5. [Crossref] [PubMed]
Cite this article as: Woodyard De Brito KC, Phelan KJ, Seitz A, Nathwani J, Janowak CF. Hierarchal clustering for the identification of distinct rib fracture patterns. Curr Chall Thorac Surg 2024;6:5.